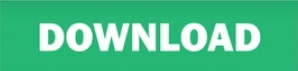
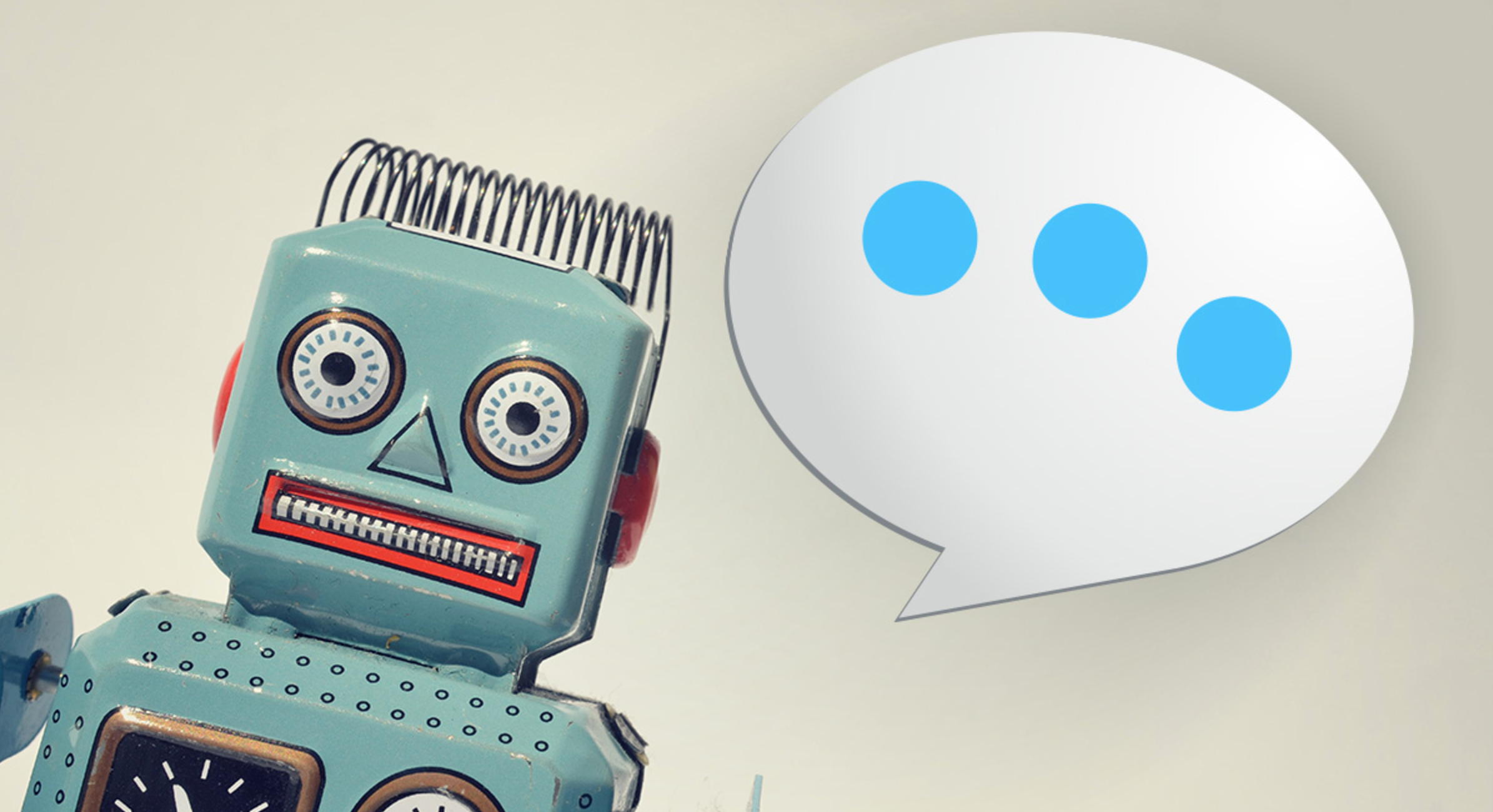
John Halamka, MD, MS, President – Mayo ClinicĬhallenge: The lack of technical know-howĭespite the advancement of artificial intelligence, most healthcare organizations lack the technical expertise to implement AI-based solutions. “The AI algorithms are only as good as the underlying data,” It can also help develop transparent algorithms, which can help train AI systems. This, in turn, helps in improving healthcare outcomes and, of course, creates more accurate databases.ĭata management and standardization can help healthcare systems across the country to eliminate siloed data. It enables healthcare providers to meticulously analyze patient records to create inferences and draw out valuable insights. Digitization with a defined set of rules with respect to the use of medical terminologies, coding values, and symbols can create unified data even across different healthcare platforms.Īnother important aspect of resolving the challenges with data is to ensure proper data management. The easiest way to do this is by ensuring the digitization of medical records. This means defining clear rules while recording patient data, using globally recognized terminologies and medical symbols, etc. The first step to resolving the challenges of AI in healthcare is to focus on standardizing medical data. So how can we solve this dilemma? Solution: Creating transparent algorithms through data management This is due to a lack of uniformity and unstructured formats. Ultimately, AI systems can barely recognize any and all available data.
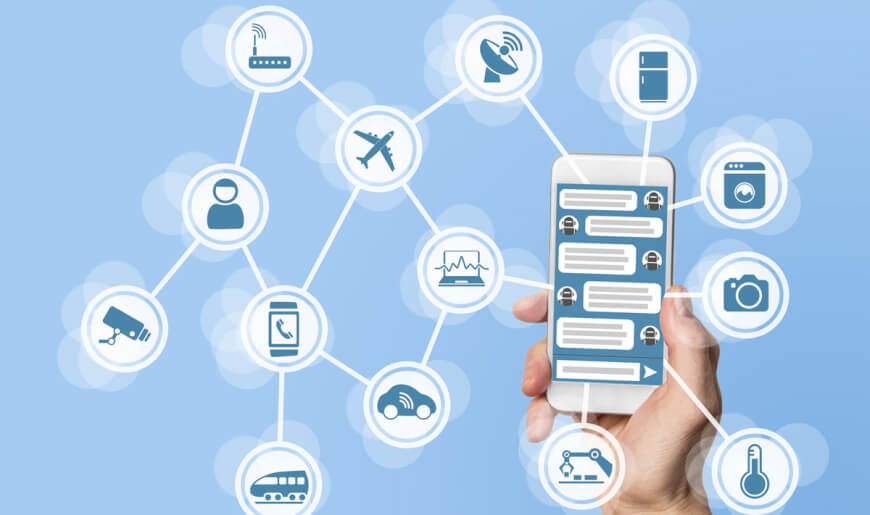
This discrepancy extends into how different healthcare systems use various terminologies, medical symbols, and more. This is a minor yet relevant example of how different diagnostic centers use different units to denote the results of the same test. On the other hand, diagnostic center B records their measurements in milligrams per deciliter (mg/dL). Now, diagnostic center A records their measurements in milligrams per liter (mg/L). Patient A goes to diagnostic center A, and Patient B goes to diagnostic center B. The challenge is to improve the data quality and standardize this data.įor instance, say there are two patients, Patient A and Patient B.īoth patients are due for their annual health checkups, and they go to two different diagnostic centers. In fact, in the US alone, over 1.2 billion clinical documents are produced annually, and this number keeps growing by 48% per year ! Now, the problem with healthcare data is not a lack thereof. There is no standardization, too many variables, and higher complexities, making it difficult to capture optimized data to train your AI systems.

This is because the healthcare approach taken by different medical institutions as well as medical staff to a single healthcare concern can be radically different. No two patient experiences are the same, which also means that the healthcare data available is erratic in nature. Unlike a number of other industries, consolidating relevant data in healthcare is no easy feat. And this is one of the biggest challenges of AI in healthcare. The four major challenges of AI in healthcare and their solutions Challenge: Consolidating valuable data for training AI systemsĪrtificial intelligence-based systems need vast amounts of data because they train on highly relevant data in order to produce accurate responses. This article will also extensively cover how these challenges can be mitigated. So then, let’s take a deep dive into four of the most pressing challenges of AI in healthcare. And this is just one hurdle.īeyond this, there are significant challenges of AI in healthcare.įortunately, with careful consideration, innovation, and strategy, healthcare systems can be equipped with the capability to overcome these challenges. The truth is that most healthcare systems would need to undergo a complete digital transformation before they could implement AI into their ecosystem. While AI has a wide range of applications in healthcare and investors waiting in line to fund the next innovative solution, AI adoption is easier said than done.Ī thorough look at the current state of healthcare systems reveals that, in reality, the adoption of AI-based solutions is still lagging behind. Investors are frantically looking for opportunities, and the pandemic has only exacerbated their appetite. The results of numerous optimistic studies, observed growth, and improvements in productivity and efficiency have definitely brought on the AI fever.
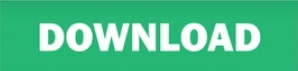